This Project is about web scraping data from the online travel agency review webpage, Trip Advisor. I had scraped close to 16k reviews online but ultimately decided to focus on the 3.5k reviews in the past two years, corresponding to the focus of the last annual report of Booking Holdings Inc.
I categorised the reviews into a database of 5 items; ratings, titles, reviews, reviewer location, and review date. I aim to gain insight into customers' sentiments toward the travel agency Agoda and learn the reasons behind their attitudes. The information can then be used as a supplement and reference for future marketing strategies. I have categorised 1-3 stars reviews as "negative reviews" and 4-5 stars reviews as "positive reviews"
Business Questions
-
How do customers feel about Agoda? Did the trend of sentiment change over time? Or was it constant?
​
​
​
​
​
​
​
​
​
​
​
-
Do different regions demonstrate different sentiments?
​
​
​
​
-
What elements or impressions spur customers to think positively or negatively toward Agoda?
Result
-
There is a clear trend of a reduction in positive reviews since 2020 Q1 when Covid-19 first started.
-
Likely due to Covid-19, the negative reviews in 2020 remained at a relatively low and stable level. However, the negative review count picked up greatly since Q3 of 2021 and remained at a high level into Q3 of 2022.
​
​
-
Reviewers that left the most amount of positive reviews are from Asian Countries such as; Thailand, Malaysia, Indonesia, Taiwan and Vietnam.
-
US and GB have an especially and alarmingly high rate of negative reviews
​
​
-
From positive reviews and positive titles. Words that appear the most frequently and are the most meaningful to me includes; easy, price, website, service, customer, booked, app, convenient
-
From negative reviews and negative titles. Words that appear the most frequently and are the most meaningful to me includes; booked, refund, customer, money, service, email, experience, cancelled
Action Summary
-
It warrants further investigation on what led to Asian countries that are 'friendly' to Agoda leaving less positive reviews over the period.
-
It warrants further investigation of the usage pattern of US and UK customers to understand the cause of their dissatisfaction.
-
Words study suggests that convenience, tech, and customer service are the dominant reasons of positive impression on Agoda
​
-
Words study suggest that "booked", "refund", and "customer service" are the dominant reasons of negative impression on Agoda.
​
-
The keywords above may serve as guidance for further investigation on how to improve customer experience and retention.
​
-
I find it interesting that contrary to positive comments, which mentioned much of fast and convenient tech such as app and website, negative comments mentioned email, which doesn't warrant timely respond, much more often instead.
DASHBOARDS (TABLEAU)

-
There is a clear trend of a reduction in positive reviews since 2020 Q1 when Covid-19 first started.
-
The negative review count picked up greatly since Q3 of 2021 and remained at a high level into Q3 of 2022.
​
-
Asian Countries such as; Thailand, Malaysia, Indonesia, Taiwan and Vietnam leave the most amount of positive reviews.
-
US and GB have an especially and alarmingly high rate of negative reviews.
* Tableau Public Server had issues with this dashboard at the time of upload. Therefore a .png file is used.
​

-
Words of interest gathered from positive reviews and titles includes; easy, price, website, service, customer, booked, app, convenient.
​
* Tableau Public Server had issues with this dashboard at the time of upload. Therefore a .png file is used.
​

-
Words of interest gathered from negative reviews and titles includes; booked, refund, customer, money, service, email, experience, cancelled.
​
* Tableau Public Server had issues with this dashboard at the time of upload. Therefore a .png file is used.
What I Can Do Better, and How?
-
There are known mistakes in data cleaning; Every time I thought I had cleaned everything, new odd cases would appear. More practice in using "regular expression" and interacting with 'HTML' tags, will improve the quality of work in the future.
​
-
Due to time constraints, I was only able to scrape and analyze reviews from one website. I would have loved to analyze a few more review websites to acquire a more comprehensive picture.
Data Cleaning Journey and Sentiment Analysis Methodology
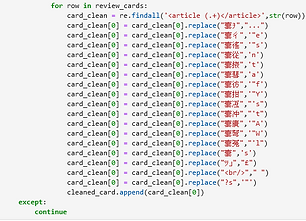

​
The data cleaning journey of this project is the most challenging thus far. Difficulties at the early stages include;
​
-
Learning to scrape data online with a new technique.
-
Figuring out how to store and manipulate HTML strings in a data frame.
-
Correcting encoding errors and leaked 'HTML' tags alike.
Everything took a considerable amount of time.
​
​
On the bright side, I learned the valuable lessons of being extra secure with the use of "regular expression". The picture on the right shows how the data frame ignored my coded regular expression's closing condition (which was set to be the next '</p>' tag after the review content) and included a long bunch of HTML code behind it.
​

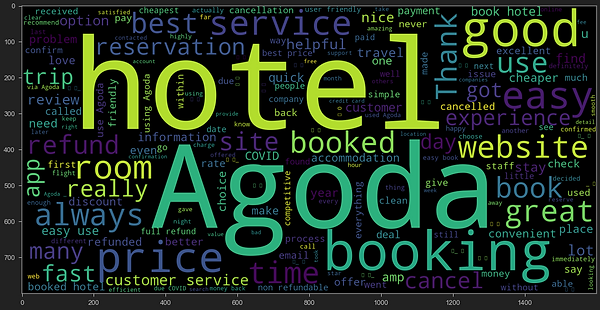
Because I am not yet employing machine learning techniques to perform sentiment analysis, I worked around the issue of identifying positive and negative sentiments by associating reviews with their star ratings.
​
Understandably, there may be outliers or users abusing the rating system. This is why as mentioned in the What I Can Do Better, and How?, section, it'd be better that I gather more reviews from multiple websites to increase my data population.
​
The word cloud creation process is relatively straightforward; after cleaning the reviews (or attempting to), they are then broken into individual characters and iterated through a punctuation checklist to remove punctuations.
​
They are then put together again, this time, iterated through a list of stopwords to remove them to the best of the python library's abilities.
​
The remaining words are then parsed into a dictionary which counts their occurrence frequency and is used to plot the word cloud.
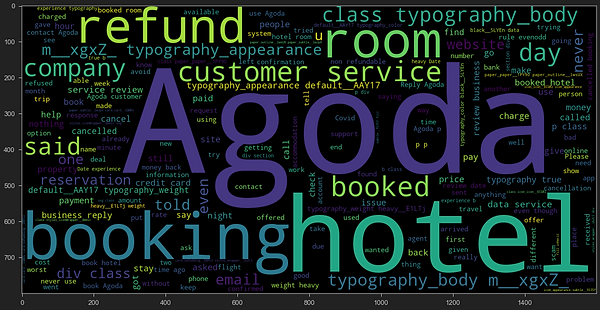